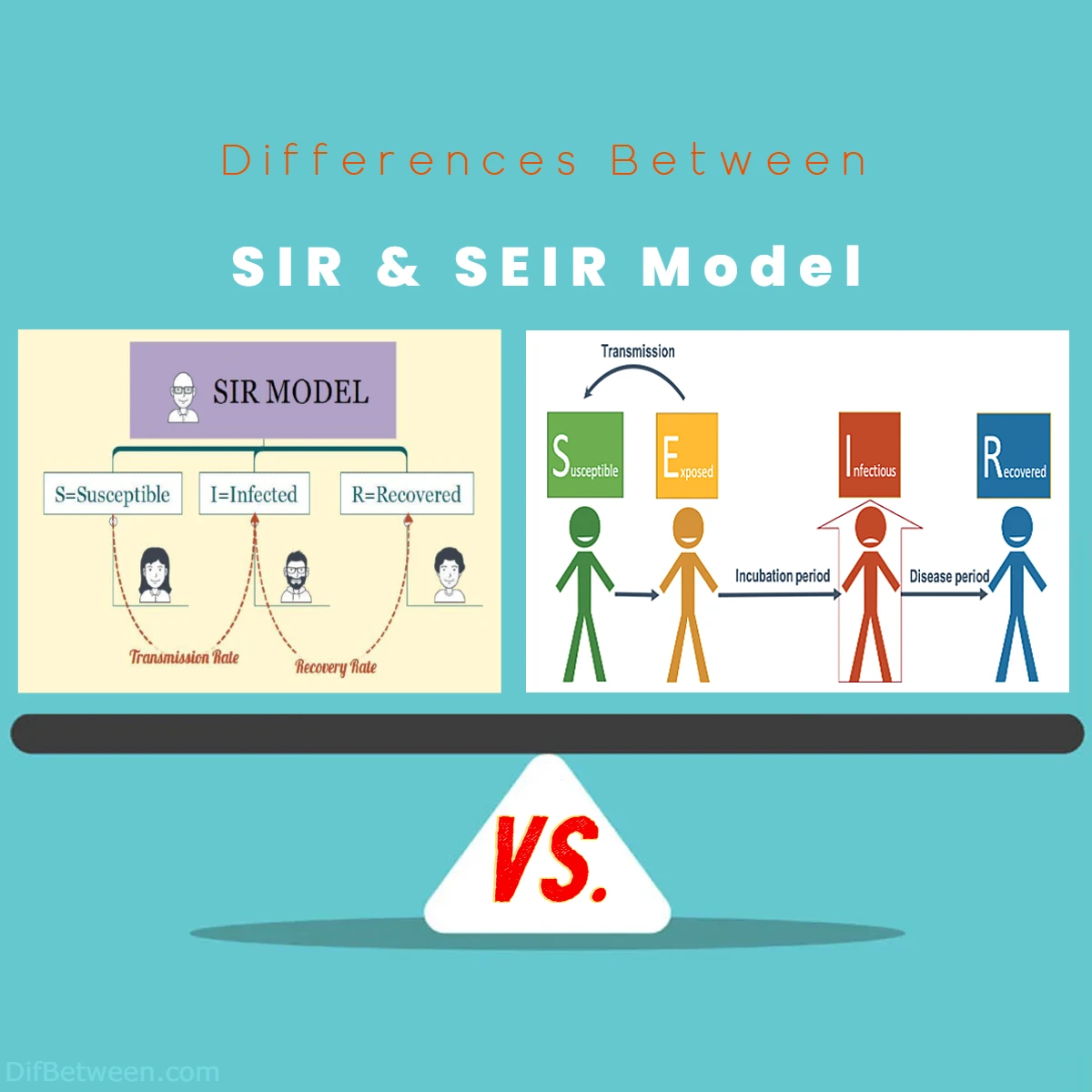
Aspect | SIR Model | SEIR Model |
---|---|---|
Compartments | S, I, R | S, E, I, R |
Incorporation of Incubation Period | No | Yes |
Disease Progression | Immediate transition from S to I | Incubation period (E) before becoming infectious |
Immunity Assumption | Permanent immunity after recovery | Permanent immunity after recovery, but adaptable |
Realism and Complexity | Simpler, less realistic for some diseases | More realistic, can capture complex transmission dynamics |
Data Fitting | Simpler, easier with limited data | More complex, benefits from extensive data |
Short-Term Predictions | Good for short-term insights | Suitable for short-term and long-term predictions |
Handling Reinfections | Does not account for reinfections | Can be adapted to model reinfections |
Complex Transmission Dynamics | Assumes homogenous mixing | Can capture variations in contact rates, heterogeneous populations, etc. |
Simulating Interventions | Basic representation of interventions | Detailed simulation of interventions and their impact |
Educational Use | Excellent for educational purposes | Useful for teaching complex epidemiological concepts |
Examples of Suitable Diseases | Seasonal flu, chickenpox | COVID-19, SARS, diseases with incubation periods |
In our quest to understand the ever-evolving landscape of infectious diseases, we’re about to embark on a journey that delves deep into two renowned mathematical models: the SIR and SEIR models. These models, like the seasoned detectives of epidemiology, unravel the mysteries of disease spread, providing critical insights that shape public health decisions. But which one should you choose for your specific needs?
Differences Between SIR and SEIR Model
The main differences between SIR and SEIR models lie in their representation of disease progression. The SIR model simplifies it by assuming immediate infectiousness upon infection, while the SEIR model incorporates an Exposed (E) compartment, capturing the crucial incubation period before individuals become infectious. This added complexity enables the SEIR model to provide more realistic insights into diseases with latent periods, such as COVID-19, making it a preferred choice for modeling such complex transmission dynamics.
1. The Foundation: SIR vs. SEIR
SIR Model
The SIR model is a classic compartmental model in epidemiology. It divides the population into three distinct compartments: Susceptible (S), Infectious (I), and Recovered (R). This model assumes that once individuals recover from the disease, they gain immunity and cannot be infected again.
Here’s a breakdown of what each compartment represents:
- Susceptible (S): Individuals in this category are vulnerable to the disease but have not been infected yet.
- Infectious (I): These are individuals who are currently infected and can transmit the disease to susceptible individuals.
- Recovered (R): People who have recovered from the disease and are immune. They cannot be infected or transmit the disease to others.
The SIR model’s basic differential equations describe the flow of individuals between these compartments over time. It’s a relatively simple model but has been effective in understanding the dynamics of diseases like the seasonal flu or chickenpox.
SEIR Model
On the other hand, the SEIR model takes a more intricate approach. It builds upon the SIR model by introducing an additional compartment, Exposed (E). The SEIR model divides the population into four compartments: Susceptible (S), Exposed (E), Infectious (I), and Recovered (R).
Here’s a closer look at each compartment:
- Susceptible (S): Same as in the SIR model, representing individuals who are vulnerable to the disease.
- Exposed (E): Individuals who have been exposed to the pathogen but are not yet infectious. This represents the incubation period when individuals have been infected but are not yet showing symptoms or capable of infecting others.
- Infectious (I): Individuals who are currently infected and can transmit the disease.
- Recovered (R): People who have recovered from the disease and are immune.
The introduction of the Exposed compartment allows the SEIR model to capture the latency period, which is crucial in diseases like COVID-19, where individuals can be infectious before showing symptoms.
2. Modeling Disease Progression: Incubation Period
SIR Model
In the SIR model, there’s no consideration for an incubation period. It simplifies the disease progression by assuming that once an individual is infected, they immediately become infectious and can transmit the disease to others. This assumption works well for diseases with short or negligible incubation periods, where individuals become infectious shortly after being infected.
While the lack of an incubation period simplifies the model, it might not accurately represent diseases with significant delays between infection and infectiousness. For instance, in the case of diseases like COVID-19, where individuals can be infectious for several days before showing symptoms, the SIR model falls short.
SEIR Model
The SEIR model excels in capturing diseases with an incubation period. By introducing the Exposed (E) compartment, it accounts for the time between infection and the onset of infectiousness. During the incubation period, individuals in the Exposed compartment cannot infect others, which aligns with the reality of many infectious diseases.
This feature of the SEIR model makes it a more suitable choice for modeling diseases like COVID-19, where asymptomatic or presymptomatic individuals can transmit the virus to others, creating challenges for disease control and prevention.
3. Realism and Complexity: SIR vs. SEIR
SIR Model
The simplicity of the SIR model is both its strength and limitation. It’s a straightforward model that is easy to understand and implement, making it a valuable tool for educational purposes and for obtaining quick insights into disease dynamics. However, its simplicity comes at the cost of realism. The SIR model assumes that individuals who recover from the disease become immune permanently, which may not hold true for all diseases. Some diseases, like the common cold or the flu, do not confer lifelong immunity.
Additionally, the SIR model does not account for demographic factors, population mixing patterns, or interventions like vaccination or quarantine. These factors can significantly impact disease spread but are not considered in the basic SIR framework.
SEIR Model
The SEIR model introduces an extra layer of complexity by considering the Exposed compartment. This added complexity allows the SEIR model to capture the subtleties of disease transmission more accurately. It can model scenarios where individuals have varying levels of infectiousness during the course of the disease, which is particularly important for diseases with complex transmission dynamics.
Moreover, the SEIR model can be extended to incorporate demographic information, vaccination, and other interventions, making it a more versatile tool for studying real-world disease scenarios. Researchers can fine-tune SEIR models to account for specific population characteristics and intervention strategies, enhancing their utility in public health planning and decision-making.
4. Data Fitting and Parameter Estimation
SIR Model
The simplicity of the SIR model has its advantages when it comes to parameter estimation and data fitting. With fewer compartments and parameters, it is often easier to estimate the model’s parameters using available data. This simplicity makes the SIR model a valuable choice when data is limited or when a quick assessment of disease dynamics is needed.
However, the drawback is that the SIR model’s simplicity may lead to less accurate fits when dealing with diseases that have more complex transmission dynamics or when data is abundant and detailed.
SEIR Model
The SEIR model’s added complexity can make parameter estimation and data fitting more challenging. With additional compartments and parameters, there is a greater need for accurate data and a larger parameter space to explore. This complexity can make it more computationally intensive and may require more advanced statistical methods for parameter estimation.
However, the SEIR model’s ability to capture incubation periods and varying levels of infectiousness can lead to more accurate fits when modeling diseases with these characteristics. When sufficient data is available, the SEIR model’s capacity to align with real-world disease dynamics can outweigh the challenges of parameter estimation.
5. Predicting Disease Outcomes: Long-Term vs. Short-Term
SIR Model
The SIR model is well-suited for short-term predictions and scenarios where disease dynamics reach equilibrium relatively quickly. It provides valuable insights into the early stages of an outbreak, such as estimating the initial growth rate and the peak of infections. However, the SIR model may not be as reliable for long-term predictions, especially when the assumption of permanent immunity does not hold.
For diseases with waning immunity or where reinfections are possible, the SIR model’s long-term predictions may deviate from reality. It’s crucial to recognize the limitations of the SIR model when using it for long-term planning.
SEIR Model
The SEIR model is better equipped to make both short-term and long-term predictions. By considering the incubation period and the possibility of reinfections (if included in the model), it can offer more realistic projections over extended periods. This makes the SEIR model particularly valuable for planning interventions and assessing the long-term impact of public health measures.
The SEIR model’s ability to capture the dynamics of infectiousness and immunity waning allows it to provide insights into the potential for future outbreaks and the effectiveness of vaccination campaigns over time.
6. Public Health Implications: Intervention Strategies
SIR Model
The SIR model can provide valuable insights into the basic reproductive number (R₀), which represents the average number of secondary infections generated by one infectious individual in a completely susceptible population. This parameter is crucial for understanding the potential for an outbreak to spread and whether interventions are needed to control it.
In the SIR model, reducing R₀ below 1 through interventions like vaccination, quarantine, or social distancing indicates that the outbreak will eventually die out. However, the model does not consider the timing of interventions or the possibility of reinfections.
SEIR Model
The SEIR model offers a more nuanced view of intervention strategies. It can help assess the timing and effectiveness of interventions during the incubation period and beyond. For diseases with the potential for reinfections or those where presymptomatic transmission is a concern, the SEIR model can highlight the importance of early and sustained intervention efforts.
Additionally, the SEIR model can simulate the impact of vaccination campaigns, taking into account factors like vaccine coverage and the duration of immunity. This makes it a valuable tool for public health officials when planning vaccination strategies to control or eliminate diseases.
7. Handling Reinfections: A Vital Consideration
SIR Model
One significant limitation of the SIR model is its inability to account for reinfections. In the SIR framework, individuals who recover from the disease are assumed to gain permanent immunity, meaning they cannot be infected again. While this assumption holds for many diseases, it is not universally true. For some infectious diseases, immunity can wane over time, or the pathogen can mutate, allowing reinfections to occur.
In reality, several diseases, including the common cold and certain types of coronaviruses, can lead to reinfections. This limitation of the SIR model becomes especially pertinent in the context of emerging diseases like COVID-19, where the duration of immunity is still a subject of ongoing research. Failure to account for the possibility of reinfections can result in overly optimistic predictions about the trajectory of the disease and the effectiveness of control measures.
SEIR Model
The SEIR model, with its added complexity and flexibility, can be adapted to address the issue of reinfections more effectively. By incorporating a variable representing the duration of immunity, the SEIR model can simulate scenarios where individuals can become susceptible to the disease again after a certain period.
This feature makes the SEIR model more suitable for diseases with uncertain immunity dynamics. Epidemiologists and public health officials can use the SEIR model to explore a range of scenarios, from short-lived immunity to lifelong protection, and assess the potential impact on disease control strategies. This adaptability is particularly valuable in the context of rapidly evolving diseases like COVID-19, where new information about immunity and reinfections continues to emerge.
8. Modeling Complex Transmission Dynamics
SIR Model
The SIR model assumes a homogenous mixing pattern within the population, meaning that every individual has an equal chance of coming into contact with any other individual. While this simplifies the model and makes it mathematically tractable, it does not reflect the real-world complexity of how diseases spread.
In reality, populations exhibit varying degrees of contact between individuals. Some individuals may have a higher risk of exposure due to their occupation, living conditions, or social behaviors. The SIR model’s assumption of homogenous mixing can lead to inaccuracies when modeling diseases with complex transmission dynamics.
SEIR Model
The SEIR model, with its ability to incorporate demographic information and contact patterns, provides a more realistic representation of disease transmission dynamics. It allows researchers to consider factors such as age, location, and occupation when modeling disease spread.
By incorporating heterogeneity into the model, the SEIR framework can better capture how diseases spread within subpopulations and communities. This is particularly important when studying diseases that disproportionately affect specific groups, such as healthcare workers or individuals in crowded living conditions. The SEIR model’s ability to account for such complexities enhances its utility in guiding targeted public health interventions.
9. Simulating Interventions and Scenarios
SIR Model
The SIR model is limited in its ability to simulate the effects of specific interventions or changes in human behavior. While it can provide valuable insights into the basic reproductive number (R₀) and the potential for an outbreak to spread, it does not allow for a detailed exploration of different intervention strategies or their timing.
In the SIR model, interventions are typically represented as a reduction in the transmission rate (the parameter β). While this simplification can yield useful insights, it does not capture the nuanced impact of interventions like testing, contact tracing, or targeted vaccination campaigns.
SEIR Model
The SEIR model’s greater flexibility makes it a powerful tool for simulating various intervention scenarios. It can incorporate detailed information about interventions, including their timing, coverage, and effectiveness. This allows researchers to assess the potential impact of specific public health measures on disease spread and control.
For instance, when modeling the COVID-19 pandemic, the SEIR model can simulate the effects of social distancing measures, mask-wearing, contact tracing, and vaccination campaigns in a more granular way. This capability helps public health officials make informed decisions about which interventions to implement and when, based on their potential to mitigate disease spread.
SIR or SEIR Model: Which One is Right Choose for You?
When it comes to modeling the spread of infectious diseases, the choice between the SIR (Susceptible-Infectious-Recovered) and SEIR (Susceptible-Exposed-Infectious-Recovered) models is a crucial decision. Both models have their unique strengths and applications, but selecting the right one depends on the specific characteristics of the disease you’re studying and the questions you aim to answer. In this guide, we’ll help you make an informed choice by highlighting scenarios where each model excels.
When to Choose the SIR Model
- Simplicity and Speed: The SIR model is an excellent choice when you need a quick, simplified analysis of disease dynamics. Its straightforward structure and fewer compartments make it computationally efficient and easier to work with, making it ideal for scenarios where you need rapid insights.
- Short-Lived Infections: If you are dealing with a disease characterized by short-lived infections and permanent immunity after recovery, the SIR model is a suitable option. It accurately captures the dynamics of diseases like the seasonal flu or chickenpox, where individuals recover and become immune for life.
- Limited Data Availability: When you have limited data on disease transmission and progression, the simplicity of the SIR model can be an advantage. It requires fewer parameters, making parameter estimation and data fitting more manageable with sparse data.
- Short-Term Predictions: For short-term predictions and assessing the initial stages of an outbreak, the SIR model can provide valuable insights into the growth rate and peak of infections. It’s particularly useful when the focus is on immediate public health responses.
- Educational Purposes: The SIR model’s simplicity makes it an excellent choice for educational purposes. It’s a valuable tool for teaching the basics of epidemiological modeling and disease spread, making complex concepts more accessible to students and stakeholders.
When to Choose the SEIR Model
- Incubation Period Matters: If the disease you’re studying has a significant incubation period during which individuals are exposed but not yet infectious, the SEIR model is the way to go. It excels at capturing this crucial phase of disease progression, making it suitable for diseases like COVID-19.
- Realistic Transmission Dynamics: When you need to model complex transmission dynamics, such as variations in contact rates, heterogeneous populations, or localized outbreaks, the SEIR model offers the flexibility to incorporate these factors. It provides a more realistic representation of how diseases spread within different subpopulations.
- Data-Rich Environments: If you have access to extensive data on disease transmission, progression, and intervention measures, the SEIR model can leverage this information effectively. Its added complexity allows for more accurate data fitting and parameter estimation when ample data is available.
- Long-Term Projections: For diseases where long-term immunity and potential reinfections are a concern, the SEIR model is a better choice. It can provide realistic projections over extended periods, helping in planning for the long-term impact of public health measures and vaccination campaigns.
- Informed Intervention Strategies: When you want to simulate and evaluate the effects of specific interventions (e.g., social distancing, contact tracing, vaccination) in detail, the SEIR model’s adaptability allows for more precise analysis. It can help you assess the timing, coverage, and effectiveness of interventions.
Making the Right Choice
In practice, epidemiologists often use a combination of models to gain a comprehensive understanding of disease dynamics. While the SIR model’s simplicity has its advantages, the SEIR model’s ability to capture complex transmission dynamics, incubation periods, and the possibility of reinfections makes it a valuable tool for many modern infectious diseases.
Ultimately, the right choice depends on your research goals, the availability of data, and the specific characteristics of the disease you’re studying. Whether you opt for the simplicity of the SIR model or the versatility of the SEIR model, both can play pivotal roles in informing evidence-based decision-making in public health and epidemiology.
FAQs
The SIR model divides the population into three compartments: Susceptible (S), Infectious (I), and Recovered (R), with an assumption of immediate infectiousness upon infection. In contrast, the SEIR model adds an Exposed (E) compartment, accounting for an incubation period before individuals become infectious.
The SIR model is suitable for diseases with short incubation periods, where individuals gain permanent immunity after recovery. It’s also handy for quick insights and educational purposes due to its simplicity.
The SEIR model is preferred when modeling diseases with significant incubation periods, like COVID-19. It’s more realistic for complex transmission dynamics, data-rich environments, and long-term projections.
Yes, the SEIR model can be adapted to consider reinfections by incorporating variables representing the duration of immunity, making it suitable for diseases with uncertain immunity dynamics.
The SEIR model is more suitable for simulating detailed intervention scenarios. It can assess the impact of interventions like social distancing, contact tracing, and vaccination in a granular manner.
Yes, epidemiologists often use a combination of models, including variants of SIR and SEIR, to gain a comprehensive understanding of disease dynamics, leveraging the strengths of each for specific aspects of analysis.
Your choice depends on the specific characteristics of the disease you’re studying, the available data, and your research goals. Simpler models like SIR may suffice for some scenarios, while SEIR offers greater flexibility for complex diseases with incubation periods.
Read More:
Contents
- Differences Between SIR and SEIR Model
- 1. The Foundation: SIR vs. SEIR
- 2. Modeling Disease Progression: Incubation Period
- 3. Realism and Complexity: SIR vs. SEIR
- 4. Data Fitting and Parameter Estimation
- 5. Predicting Disease Outcomes: Long-Term vs. Short-Term
- 6. Public Health Implications: Intervention Strategies
- 7. Handling Reinfections: A Vital Consideration
- 8. Modeling Complex Transmission Dynamics
- 9. Simulating Interventions and Scenarios
- SIR or SEIR Model: Which One is Right Choose for You?
- FAQs